
Posted: February 7, 2024 10:01 AM Updated: February 7, 2024 10:01 AM

Correction and fact check date: February 7, 2024, 10:01 AM
briefly
ML is transforming analytics, detection, personalization, and automation, blurring the lines between traditional BI and advanced analytics.
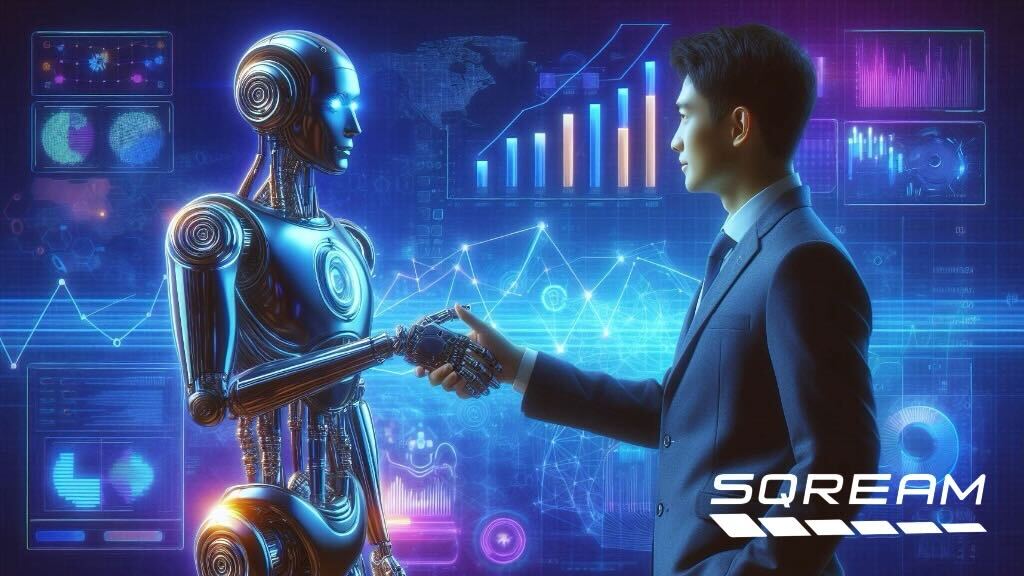
The value of any tool depends on how you use it to get results. Likewise, companies understand that success depends not on the data they have, but on how they utilize it.
As the size and importance of data grows rapidly, the business intelligence (BI) and data analytics landscape is undergoing permanent change. As traditional analytics become more dynamic and powerful, some see this as the end of BI as we know it.
This change is largely driven by machine learning (ML), a self-improving data analysis process that is playing an increasingly pivotal role in nearly every aspect of business operations. Companies using BI for data analysis increasingly need machine learning capabilities.
Here’s what data managers and enterprises need to know to stay ahead of the machine learning curve.
The traditional role of data analytics
Long synonymous with data analytics, business intelligence typically involves dashboards and reports gleaned from data stored in a data warehouse or lakehouse that helps organizations understand past trends and patterns.
These traditional approaches are no longer sufficient to accommodate today’s data deluge. There is too much data for a simple dashboard readout or analytics report to fully reflect the insights of a particular data set.
BI technologies use data to track trends over time and gain valuable insights that might otherwise be overlooked, but they typically analyze data as isolated packages of information. Therefore, human analysts and relevant decision makers must form predictions based on that information.
The Rise of Machine Learning
Although ML is still a relatively new addition to the enterprise technology stack, it has become a major driver driving data analytics forward. ML along with Generative AI are so trendy that business executives are often forcing data managers to implement ML before use cases are identified.
Instead of passively evaluating received data, as is the case with BI, machine learning enables systems to actively learn from data, make independent predictions, and adapt to new information accordingly.
Here are some properties of ML that can fundamentally transform the business analytics landscape:
- Predictive Analytics – ML allows companies to do more than simply understand historical data. Because ML can predict future outcomes more accurately. By identifying patterns and relationships within a data set, ML models can make predictions that help decision makers proactively shape strategies, optimize resource allocation, and mitigate potential risks.
- Real-time analysis – Unlike regular reports from traditional BI, ML-based analytics provides real-time insights. These real-time analytics enable organizations to quickly respond to changing circumstances, capitalize on new opportunities, and make informed decisions, creating a more agile and adaptive business environment.
- Anomaly Detection – ML algorithms automatically identify outliers and outliers in data, helping organizations detect fraud, errors, and security breaches faster than ever before. By quickly detecting and flagging anomalies, ML increases the effectiveness of risk management and helps you take proactive steps to protect against potential threats.
- automation – ML can automate repetitive tasks, reducing the manual work required to analyze data. By learning from historical data and patterns, ML algorithms can take care of mundane and time-consuming tasks, freeing employees to focus on more strategic and creative tasks.
Blurry boundaries between BI and ML
As more companies adopt ML for analytics purposes, the differences between traditional data analytics and ML-based analytics become increasingly clear.
Many activities traditionally associated with BI, such as reporting and dashboard creation, now rely on ML-based algorithms for more accurate and actionable insights that are adjusted in real time. For example, instead of generating reports manually, companies can use ML algorithms to automatically generate reports to highlight the most relevant information and historical trends while also predicting how these trends will change in the future.
These changes blur the lines between BI and ML, emphasizing how the practice of analytics is broader than any specific tool or approach. Instead, it is evolving into a dynamic and predictable field. There’s a reason some people have started calling ML “advanced analytics.”
BI Ribbon
As ML becomes a more common and widely used tool, business intelligence is no longer limited to analyzing historical data. Instead, ML transforms data analytics to fundamentally reshape business environments.
To remain competitive and make data-driven decisions, organizations must adapt to evolving paradigms and integrate machine learning into their data analytics processes. The pace of this adoption process will vary from company to company, but all data-dependent organizations will invest in appropriate ML technologies, upskill their employees, and create a data-driven culture that values insights derived from ML.
The rise of ML does not mean the “death” of BI if BI is perceived as a process or approach to business rather than a tool. Instead, it means a rebirth, a transition to the beginning of a more intelligent, advanced and automated future.
disclaimer
In accordance with the Trust Project Guidelines, the information provided on these pages is not intended and should not be construed as legal, tax, investment, financial or any other form of advice. It is important to invest only what you can afford to lose and, when in doubt, seek independent financial advice. We recommend that you refer to the Terms of Use and help and support pages provided by the publisher or advertiser for more information. Although MetaversePost is committed to accurate and unbiased reporting, market conditions may change without notice.
